Knowledge work often involves unfamiliar experiences with goal-relevant percepts and rules to learn new information and create novel concepts. Attention is a prerequisite for practical knowledge work and generally requires significant cognitive effort to focus and sustain over time.
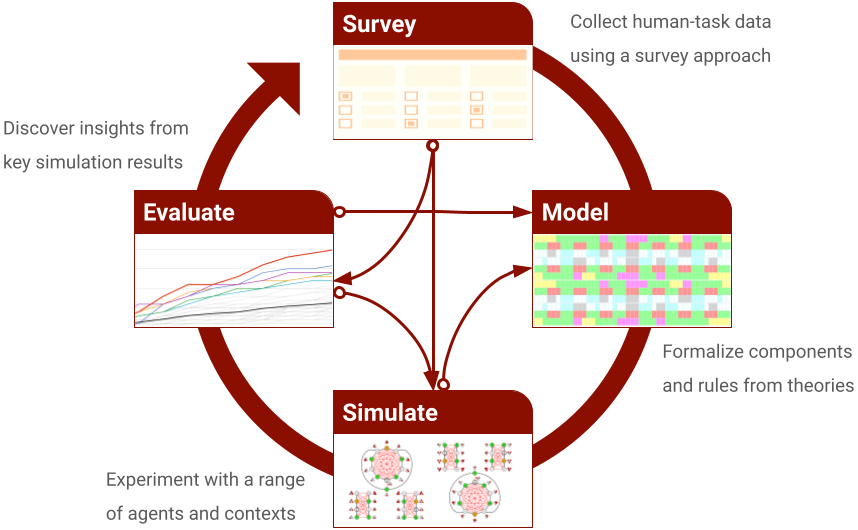
The challenge of knowledge work is driven by the novelty and complexity of the task and correlates with the subjective enjoyment of the activity. Humans perceive flow as the optimal state of awareness during which task performance maximizes and self-awareness minimizes. Research studies on flow to date have a significant disagreement regarding what flow is and how to measure it.
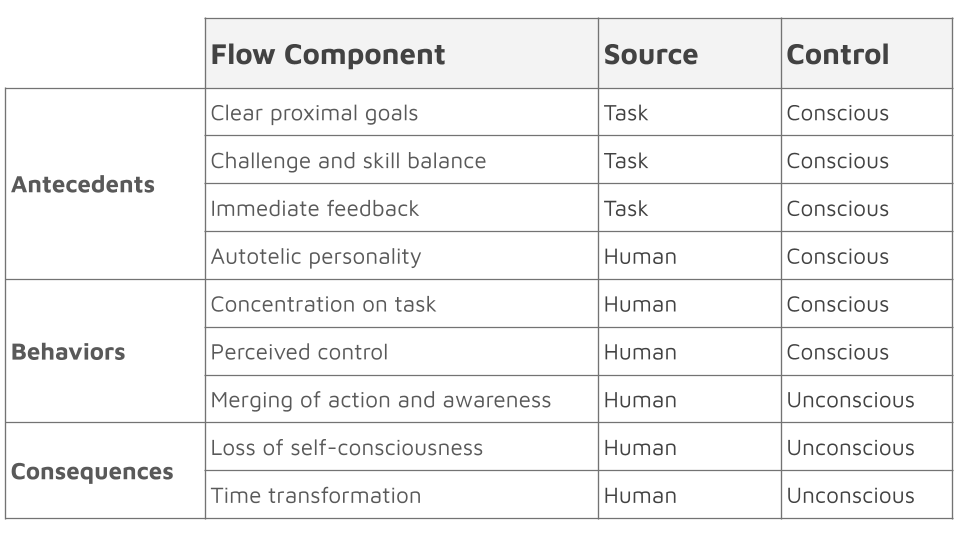
This paper proposes a formal human-task-context performance model of flow that integrates attention, surprise, and enjoyment to measure flow using active inference and Markov Decision Processes. We administered a cross-sectional questionnaire with knowledge workers to obtain priors for our Bayesian model, capturing evidence about the flow components to make inferences about knowledge work performance.
Our hypothesis states that when the human knowledge worker experiences flow at work, their ability to focus and sustain attention on the task is maximized, which minimizes their perceived ambiguity and stress, thereby resulting in effortless attention, which perpetuates flow.